STATE OF GENAI MONETIZATION RESEARCH REPORT
A Glimpse into the Future of GenAI Pricing Metrics and Models
A data-driven analysis of offerings from 70+ companies
Authored by: Michael Mansard, EMEA Chair of the Subscribed Institute
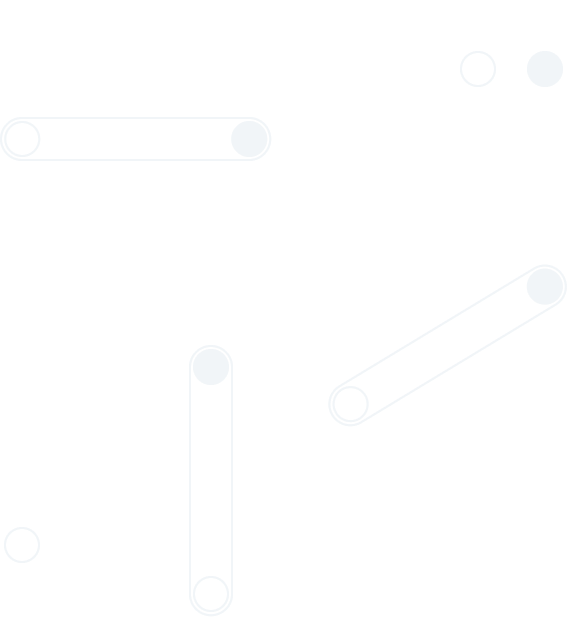
Methodology: In this ongoing data-driven analysis, we examine the monetization strategies of over 70 companies with GenAI offers. Research, analysis, and recommendations based on publicly available information from press releases, articles, and vendor websites as of April 2024.
Explore the insights

As artificial intelligence (AI) and Generative AI (GenAI) continue to revolutionize multiple industries, effective monetization strategies are a vital ingredient for companies to keep up with adoption and sustain growth. Balancing innovation with sustainable revenue models, while also ensuring customer satisfaction and market competitiveness is paramount.
Throughout this series, we’ve examined over 70 GenAI offers in order to identify key strategies for balancing the major factors involved in the launch of a GenAI offer: overcoming the monumental costs, driving adoption, and capturing value.
We’ve also looked at the leading trends in how these GenAI offers are being positioned and packaged, shining a light on the benefits and challenges of the various monetization strategy decisions companies currently face.
In this article, we’ll explore the range of GenAI pricing metrics and models, highlighting the accelerating shift towards usage-based (also known as consumption-based) pricing metrics and value-focused strategies, such as hybrid models, to better align prices with the actual value delivered to customers, while also hedging against the costs.
We find that traditional seat- or user-based pricing, though common, is no longer sufficient to capture the unique and dynamic value of GenAI. In fact, the answer likely isn’t to be found in any singular pricing model. Instead, as a handful of visionaries in the space have already discovered, adopting a Total Monetization approach for GenAI requires a continuous journey of data collection and experimentation in order to arrive at an end-to-end strategy (Exhibit 1).
Exhibit 1: Learn and iterate to achieve GenAI Total Monetization
“We’re firmly in the adoption foot race, but as consumption usage and value patterns emerge, we would expect the pricing models to continue to evolve.”
– Colin Carroll, Pricing and Commercial Excellence at PwC
Section 1: Learning from GenAI pricing metric trends
A pricing or value metric is a quantifiable measure that forms the basis for pricing a product or service. Selecting the appropriate pricing or value metric is crucial because, ideally, it should align the price of the service with the value it delivers. This helps ensure fairness and transparency in pricing, which can significantly affect customer satisfaction and retention.
As seen below, these units or metrics range from declarative and committal, such as a “number of users,” to usage-based, such as “number of chat responses” (Exhibit 2).
Exhibit 2: GenAI pricing metrics fall across a spectrum
Spectrum of pricing metric options:
- Flat (no metric): Subscription models that do not charge based on a metric. Given that these products are typically targeted towards consumers and SMBs more than enterprises, this approach makes sense.
- Inputs: Focus on the resources required, or inputs, to run GenAI processes, such as the number of users or seats. For example, GitHub charges per user for its Copilot tool.
- Activities: Based on specific actions that contribute to an output, such as the number of GenAI queries or GPU hours used. Companies like C3.AI, for example, charge per virtual CPU (vCPU) hour. Microsoft’s Copilot for Security is charged per security computing unit hour.
- Outputs: Relate to the tangible outputs generated by GenAI activities, such as the number of emails or images generated. Adobe Firefly, an AI-powered image generator, meters usage per number of images created.
- Outcomes: Measure the business impact achieved through GenAI, such as the percentage of successful customer interactions. Chargeflow charges per successful chargeback settled in the customer’s favor.
As Exhibit 2 shows, a small percentage of the offers in our study rely on flat-fee pricing—not using a metric at all. However, it seems that most companies recognize that not employing a pricing metric is growth prohibitive. Studies show that companies who use a metric grow 30% faster than those who don’t. Selecting the right pricing metric is critical as it ensures fairness, transparency, good value realization, and customer satisfaction and retention.
And while nearly 50% of offers are currently in the input-based category (Exhibit 2), the situation is a bit more complex. As we’ll discuss in Section 2, some of these seat-based offers are already infusing some level of usage-based metering and thresholding on top of user-based pricing.
But first, let’s take a closer look at what our research reveals about how companies are currently using each of these 4 metric types to track and monetize their GenAI offers.
Inputs
Our research has uncovered that input-based metrics, such as per user or per seat, are the most prevalent for GenAI. This is because these well-established SaaS pricing metrics offer a familiar and easy way to quickly take new offers, like GenAI, to market. As a result, input-based metrics are used by more than half of the 70+ offers we analyzed—especially GenAI Applications (Exhibit 3). Notable examples include the Salesforce Einstein add-on, which is priced at $70/month per user and ChatGPT Team at $25/month per user.
Exhibit 3: Pricing metric distribution for GenAI applications and models*
*For more information on the GenAI value chain, check out our previous installments: Monetizing GenAI: Why most SaaS companies are missing out and The Leading Packaging Models for GenAI.
While this tried and true method works well in the early launch stage and does help foster adoption, the novelty of GenAI technologies is forcing product leaders and executives alike to step back and reevaluate pricing best practices. In fact, early data indicates that using seat-based metrics in isolation can result in an adverse effect for GenAI offers.
Here’s why. It’s like the old gym membership paradox or the ill-fated Delta Unlimited Flight Pass—a highly active user, who should extract more value from the service, is charged the same as a less active user. In the end, these more engaged users drive lower margins, but user-based metrics cannot track or accommodate for this variation in value delivery and costs. This means that providers are not incentivized to cater to these users, who should be their bread and butter.
This misalignment could have disastrous consequences. Microsoft’s GitHub Copilot is already reportedly feeling this pain, losing an average of $20 per user each month, and sometimes as much as $80/month for active users. This provides a perfect illustration of why, in the enterprise setting, buying chatbot or copilot licenses for every single employee likely isn’t cost effective at this point in time.
In the long term, vendors anchoring on input-based metrics alone will suffer from more limited agility and profitability, as rigid pricing restricts value capture and becomes a bottleneck for future revenue growth. With this in mind, we predict that user-based pricing will only be a transitional model for the majority of GenAI offers. In fact, an ever increasing number of companies are already moving to value-based models that leverage usage-based metrics—in addition to user-based metrics.
Let’s take a closer look at more effective value-based approaches.
A transition from user-based to usage-based metrics
Usage-based pricing, also known as consumption or metered pricing, is a model that charges customers based on their actual usage of a product or service. This approach has gained significant traction in recent years, particularly in industries such as telecommunications, utilities, SaaS—and now—AI.
With the recognition that flat or user-based pricing alone may not be adequate for GenAI, many companies are beginning to employ usage metrics—such as activities, outputs, and outcomes—to measure and set thresholds on GenAI usage.
Benefits of usage-based metrics:
- Encourages adoption: Lower initial costs can drive higher adoption rates, as customers only pay for what they use.
- Flexibility: Customers can start with minimal commitment and scale up their usage as they see value, providing a low-risk entry point.
- Customer retention: Continuous alignment of pricing with actual activities, outputs, or outcomes helps maintain customer satisfaction and loyalty, as they pay in proportion to their usage.
- Usage visibility and transparency: Real-time tracking of activities allows customers to monitor their usage and spending, increasing transparency as well as customer satisfaction and control.
- Incentivizes quality: Providers are motivated to improve the quality and efficiency of their GenAI to maximize outputs or outcomes, benefiting both parties.
- Innovation and experimentation: Providers can test and iterate on different activity-based pricing models to find the optimal structure that maximizes both customer satisfaction and revenue.
- Enhanced competitive advantage: Providers offering usage-based pricing, especially outcomes, may stand out in the market, attracting customers looking for guaranteed results.
- Aligns with cost: Even though it is usually not advisable to price based on costs for intangible services such as GenAI, usage-based metrics can potentially help mitigate the associated high costs and lower profit margins.
Challenges of usage-based metrics:
- Potential for complexity: When usage-based metrics are used in isolation, without some level of commitment, managing and predicting costs and revenue can be more challenging for both providers and customers.
- Billing complications: The billing process can become more complex, leading to potential disputes over charges. Real-time customer usage data visibility becomes vital—across the business.
- Risky for providers: With outcome-based metrics in particular, there’s a high risk if the desired results are not achieved or are difficult to measure. Both the vendor and the customer will need to align on and agree to a set definition for what constitutes a successful outcome.
- Customer overcommitment: If there is some level of commitment, there’s a risk that customers might commit to more usage than they can sustain, especially for new and developing innovations like GenAI services, where use cases and value are still being explored.
Activities and outputs
Activity-based and output-based metrics are already the go-to approach for GenAI offers in the model layer of the value chain (Exhibit 3). Yet in those cases, they are set mostly in a “cost plus” spirit, in other words, aligning the cost model to the revenue model. This is likely because it’s the typical way for IaaS and PaaS providers (Azure, Google Cloud Platform, AWS, etc.) to sell, and they’re addressing similar buyers. And users themselves are not directly accessing GenAI models per se; instead, the consumption originates from another system at the application level.
Yet, each and every “token” of activity or output has a very different value, depending on the use case and context that is run in the application layer (Exhibit 4).
Exhibit 4: Activity-based and Output-based metric examples
Outcomes
Suitable for GenAI solutions that deliver specific business results, outcome-based metrics underscore the value of GenAI by directly linking the price to the business outcomes it helps achieve. This type of metric works well in industries focused on performance metrics like sales and marketing automation or financial forecasting.
Well designed outcome-based models can even further optimize value capture, as they are customer-centric metrics by their very nature, aligning with customers’ strategic objectives, such as achieving customer service resolutions or chargeback settlements (Exhibit 5). Such metrics do require a mutual understanding of value between the provider and the user. To do this, vendors will need time to uncover the underlying cost and value drivers of the process or workflow covered by the solution for both parties.
This is why early adopters of outcome-based models are usually found in customer service or support use cases, where the cost to reach a resolution is already known. Intercom, an AI customer service solution, is a perfect example of this (Exhibit 5).
Exhibit 5: Outcome-based metric examples
Although outcome-based metrics are only used by 6% of the offers in our study (Exhibit 3), throughout this series, we’ve predicted an increase in such metrics in the coming months and years, as companies continue iterating on their monetization strategies to optimize value realization.
While industry-specific GenAI solutions like Harvey.ai and Hippocratic.ai don’t appear to be using outcome-based metrics at this time, there is a strong argument to be made for the growth potential of such a strategy.
In our previous installment, we also predicted that such solutions would shift towards persona- or function-based packaging over time for similar reasons—both strategies aim to align pricing and packaging with the value the customer actually receives.
The following attributes can be predictors of the success of GenAI products in moving towards a more value or outcome-oriented model, thus capturing a higher value premium of the solution.
Uniqueness of underlying data set | Exclusivity, depth, and rarity of the data used to train the GenAI model, especially if the data set provides unique insights or competitive advantages. |
Specialization | Specialization in a specific domain or use case, addressing clear problems with high precision, making it more valuable to targeted customers. |
Attributability of value creation | Ability for the solution to be directly linked to quantifiable impact in terms of value creation and continuous return on investment (revenue growth, cost savings…). |
Time-to-value | Speed with which customers can deploy the solution and begin realizing tangible benefits, as quick wins support investment justification and prioritization. |
Scalability of solution | Ease with which the solution can be scaled elastically to accommodate growth in data, users, or complexity, affecting long-term value and adaptability. |
Regulatory and local compliance | Adherence to relevant regulations and data privacy measures, especially niche or local ones, minimizing legal risks for customers. |
Position in the GenAI value-chain | Criticality within the broader GenAI ecosystem. |
As companies move towards outcome-based metrics, this will require more data and understanding of jobs-to-done. This will lead to hypersegmentation of offers, using a mix of carefully selected customer-centric metrics.
Luckily, existing data already proves the growth potential of this approach. Subscribed Institute research shows that companies that have 3-5 charge models, where each charge model contributes at least 10% of total revenue, perform the best when it comes to year-over-year revenue growth, average revenue per account (ARPA) growth, and average monthly churn rate (Exhibit 6). In the next section, we’ll dive into the proven pricing models that can help achieve this growth.
Exhibit 6: Businesses with more charge models perform better
Section 2: Combining pricing metrics into models for GenAI
Now that we’ve covered pricing metrics, let’s take a look at the types of commercial levers and pricing models—or mix of models—companies are using to take their GenAI offers to market.
Nearly half of the GenAI offers we studied employ a committed recurring model, often using an input-based metric (Exhibit 7). In these instances, the offer usually includes unlimited use of GenAI, often with a fair use policy (NotionAI is an example of this). Or, the committed recurring plan may include a number of GenAI interactions or credits that can be increased by moving to a higher tier. Both HeyGen and Anaconda Assistant appear to be adopting this tactic.
If we dig a little deeper, we can see that vendors are already employing a range of pricing models.
Combining the best of both worlds with hybrid models
While most GenAI applications initially adopt flat or user-based pricing due to the simplicity and familiarity of such models, an ever increasing number of visionaries are adopting hybrid pricing models. For GenAI, where revenue growth is currently hard to come by, this is a great strategy. In fact, research shows that SaaS companies employing these models achieve faster growth than their peers.
However, as we discussed in the first installment in this series, the ultimate aim of GenAI monetization is not merely competitive pricing, but creating true value. Despite the pressures to cut costs and automate, the path forward is about aligning costs closely with value delivery.
Hybrid pricing models can help achieve this alignment by integrating a mix of pricing metrics, such as input-based and outcome-based models, optimizing value capture and driving revenue growth (Exhibit 7).
Whereas seat-based pricing alone cannot scale with the changing demands and capabilities of GenAI, layering usage on top can help improve scalability. In fact, over 50% of the offers in our study are already employing some type of hybrid model, often combining pre-commitment or recurring commitments with some level of usage-based pricing (Exhibit 7).
Exhibit 7: GenAI pricing metrics and models
Unlike simple recurring charges that are usually charged in advance, these hybrid models come with more complexity. Hybrid pricing models range from predominantly pay-as-you-go consumption, to pre-pay with drawdown, or pre-pay a minimum commitment and pay for overages.
Usage- or consumption-based hybrid models are already widespread in the GenAI model layer of the value chain. These offers, such as MistralAI, often employ output-oriented metrics such as pay-per-token. While Chargeflow and a few other pioneers are already employing outcome-oriented metrics, such as % of chargeback value settled in your favor.
Committed recurring with overage models usually take the form of input-based pricing combined with a set number of generative credits, some form of usage thresholding, and the option to purchase more upon overage.
Usage thresholding can help companies overcome the monumental costs of GenAI. At the moment, we see three variations:
- Quantity thresholding: the number of interactions per period are limited.
- Speed thresholding: the speed of processing is limited.
- Quality thresholding: the quality of the model used or the generated output is inferior.
Adobe Firefly wisely combines these thresholds, given that users can still continue using the service after depleting their allotted credits, however the processing time may be slower, tasks may be limited, or image quality may be reduced.
Exhibit 8: A spectrum of metrics used to build a spectrum of hybrid models
Lastly, there are many metric mixing options for hybrid models, ranging from a tiering model (similar to tax brackets) to a high watermark model, where charges are based on the highest level of usage or service consumption within a specific period A non exhaustive visual list is provided in Exhibit 8. To summarize, this just adds one last dimension in the immense, if not infinite, scope of pricing and packaging possibilities.
Hybrid models do require a strong discipline in customer usage metering to ensure revenue assurance, given the risks of revenue leakage when managing complex models. But with the right strategies and tools, these models may provide the growth, scalability, and flexibility that GenAI startups and SaaS companies alike require.
Reasons to choose hybrid models for GenAI:
- Accelerated annual recurring revenue (ARR) growth: Research shows that SaaS companies using hybrid models experience faster growth than those relying solely on flat or user-based pricing. By integrating both subscription and consumption-based elements, businesses can optimize value capture and drive significant revenue growth.
- Scalability and flexibility: Hybrid models offer the flexibility to scale with the evolving demands and capabilities of GenAI. While traditional seat-based pricing fails to accommodate changing usage patterns, adding a usage-based component helps meet diverse customer needs.
- Higher customer retention and average revenue per account (ARPA): By combining committed recurring payments with the right level of usage-based charges, hybrid models strike a balance that leads to higher ARPA and better retention rates.
- Rising preference for usage-based pricing: Both consumers and tech buyers show a strong preference for usage-based pricing, particularly in the AI and GenAI sectors.
- Optimal value capture: Using a mix of pricing metrics, such as user-based and outcome-based units, hybrid models ensure that pricing aligns more closely with the actual value delivered to customers, which is absolutely vital for the long term success of GenAI offers.
- Improved revenue assurance: Hybrid models require robust customer usage metering, which helps minimize the risks of revenue leakage. This precise tracking and billing of usage fosters a disciplined revenue management approach.
- Enhanced predictability: Customers benefit from the predictability of committed recurring payments and the flexibility of usage-based charges for overages. This dual approach accommodates varied budget preferences, making it easier for customers to manage costs and scale their usage as needed.
- Encouragement of incremental commitments: Hybrid models allow customers to start with minimal commitments and gradually increase their investment as they recognize more value. This phased approach enables mutual value discovery and stronger long-term engagements.
- Support for diverse use cases: These models cater to a wide range of metrics, from output-oriented (e.g., pay-per-tokens) to outcome-oriented (e.g., percentage of chargebacks settled). This versatility makes hybrid pricing suitable for various GenAI applications.
Embracing flexible and customer-centric strategies for sustainable GenAI monetization
The shift towards usage-based metrics and hybrid pricing models marks a critical departure from traditional seat- or user-based pricing, which no longer adequately captures GenAI’s unique value. Adopting a Total Monetization approach, companies must continuously gather data and experiment with diverse pricing metrics to align costs with customer value and ensure competitiveness and satisfaction.
The future of GenAI monetization lies in a flexible, data-driven approach that balances cost efficiency with value delivery, ensuring long-term success and growth in the GenAI-driven marketplace.
Key takeaways
- The user-based paradigm will be unsustainable for most GenAI offers, so becoming familiar and experimenting with the spectrum of value metrics is paramount.
- Although outcome-based metrics may be the ultimate destination for some companies, getting there will require a journey, and even then, not all offers are ideal candidates for this approach.
- As companies fine tune their approach to more closely align pricing with value, it will require increased data collection, more customer centricity, experimentation, and hypersegmentation.
This article contains forward-looking statements that involve a number of risks, uncertainties and assumptions, including but not limited to statements regarding the expected benefits and drawbacks of releases of GenAI solutions with different pricing metrics and models. Any statements that are not statements of historical fact may be deemed to be forward-looking statements. Actual results could differ materially from those stated or implied in forward-looking statements due to a number of factors. The forward-looking statements in this article are based on current expectations as of the date of this article and Zuora undertakes no obligation to update or revise any forward-looking statements, whether as a result of new information, future events or otherwise. To the extent that this press release also includes market data and certain other statistical information, that information is based on estimates, forecasts, projections, or similar methodologies, and is inherently subject to uncertainties, and actual events or circumstances may differ materially from events and circumstances reflected in this information. As stated in the article, the recommendations are a foundational guide, but not intended to be predictions of the future or accurate advice.
Leading strategies for monetizing GenAI
The 4 GenAI monetization avenues
Explore the leading positioning strategies of the pioneers in the GenAI monetization frontier—and the pros and cons of each.
Packaging models for GenAI
Discover how companies are packaging their GenAI offers today and learn the strategies that will set your business up for success.
Learn more about the author
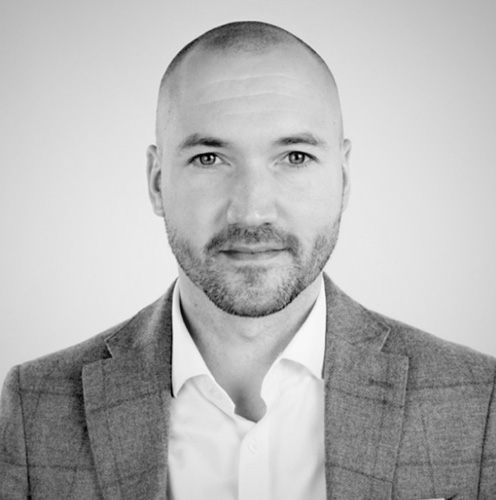
EMEA Chair, the Subscribed Institute
Principal Director of Subscription Strategy, Zuora
The Subscribed Institute
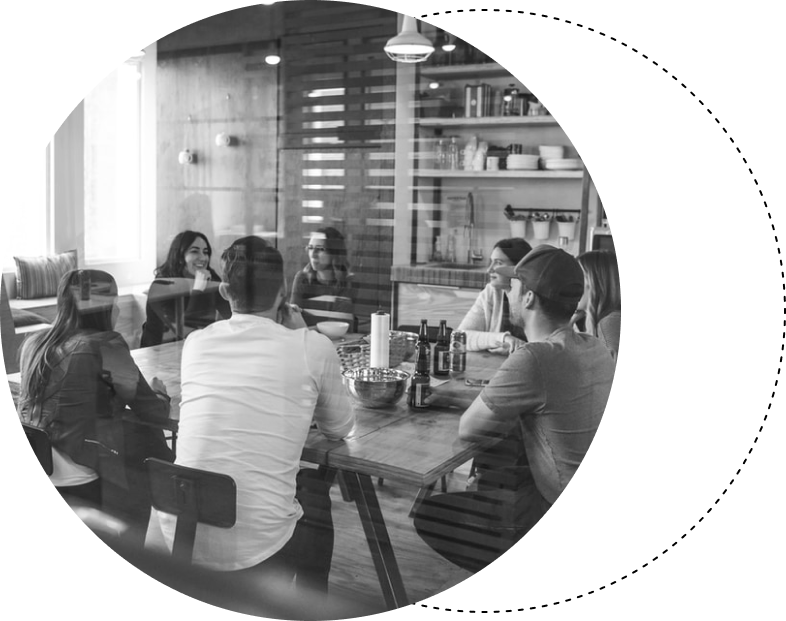