State of GenAI Monetization Research Report
Monetizing GenAI: Why most SaaS companies are missing out, and how to fix it
Authored by: Michael Mansard, EMEA Chair of the Subscribed Institute
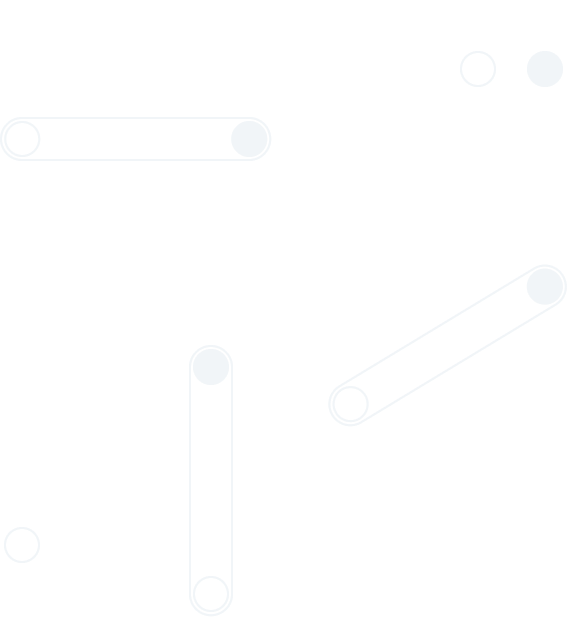
Methodology: In this ongoing data-driven analysis, we examine the monetization strategies of over 70 companies with GenAI offers. Research, analysis, and recommendations based on publicly available information from press releases, articles, and vendor websites as of April 2024.
Explore the insights

The year 2023 will likely be remembered as the year generative AI (GenAI) graduated from a promising technology to a mainstream innovation. As companies race to push out new GenAI products, end users are exploring the potential in their personal and professional lives. We’ve seen great wins and we’ve seen some flops. The pace of innovation is fast and with that comes an emergence of new business models.
While there are standalone AI offerings, established companies are also injecting generative AI into their product suites. In fact, as reported in 2023, over 3 in 4 SaaS companies launched or are currently developing a GenAI offering.
Despite the buzz, only 15% of those same companies have monetized their AI solution. In order to move quickly to market, many companies are announcing or launching their products before thinking through their long-term monetization strategy. Some even choose to forego monetization altogether at time of launch.
When we dive into examples later in this series, it turns out that many of those companies are simply defaulting to simple pricing models rather than finding the best, future-proof monetization strategies for these new generative AI offerings. With the high costs and lower profit margins associated with GenAI, this could prove to be disastrous.
And while price wars between competing offers (such as ChatGPT Plus, Google One AI Premium, and Amazon Q Business) may create the illusion that fine tuning price points is all that’s needed for successful GenAI monetization, the issue is much more complex.
To actually have staying power in this increasingly competitive, evolving landscape, modern businesses could benefit from equally agile approaches. It requires iteration on monetization strategies to generate revenue from their GenAI offerings. And by continually evolving pricing to generate revenue and growth with the changing demands of customers, companies can achieve what we call Total Monetization.
As we witness the birth of this new market, 2024 is the year where GenAI monetization truly begins. The winners in this space won’t be the ones who simply “give it away for free,” but the ones that adopt Total Monetization strategies.
What are these strategies and how can companies employ them to help align and adapt GenAI monetization to scale with changing customer demands?
At first glance, you might assume there is some level of homogeneity in the current approach to monetization. However, our analysis of over 70 GenAI offers reveals a rich tapestry of strategies developing in real time.
In this series, we will document and explore the evolving state of the GenAI market, starting with the cost, adoption, value triangle—and what this can reveal about the reason monetizing GenAI is still a missed opportunity for so many companies.
Explore the rest of the series on GenAI monetization:
Most SaaS companies are missing the opportunity to monetize GenAI
The swift adoption of GenAI by businesses and individuals is the result of its utility-driven design, user-centric solutions, and simplified interfaces. However, due to the rapidly shifting nature of this technology and market, clear guidance for GenAI monetization best practices is lagging behind.
Testing and iterating on monetization strategies for SaaS has always been a fine art, but for GenAI, moving quickly and learning from the current leaders is absolutely vital for success. If monetization is an afterthought, failure is quite likely. With GenAI gross profit margins being, on average, 10 to 30 pts lower than SaaS, profitability is challenging right from the start.
For companies developing and launching GenAI solutions, landing on the right monetization strategy for GenAI will require an ongoing balancing exercise. Our research indicates that companies should continuously explore and aim for equilibrium among three key monetization focus areas:
- Cost-oriented: This approach is simple to set up while securing margin levels, but is decorrelated from customer value. This means money is often either left on the table, or you end up overcharging.
- Adoption-oriented: Makes it easy for customers to onboard and do business with you, but at the expense of profit margins (especially given specific GenAI cost economics as compared to SaaS that we will discuss).
- Value-oriented: Ensures alignment between value creation and value capture, but requires the strategy and tools to directly measure and demonstrate value. This approach should be the long-term target for most companies.
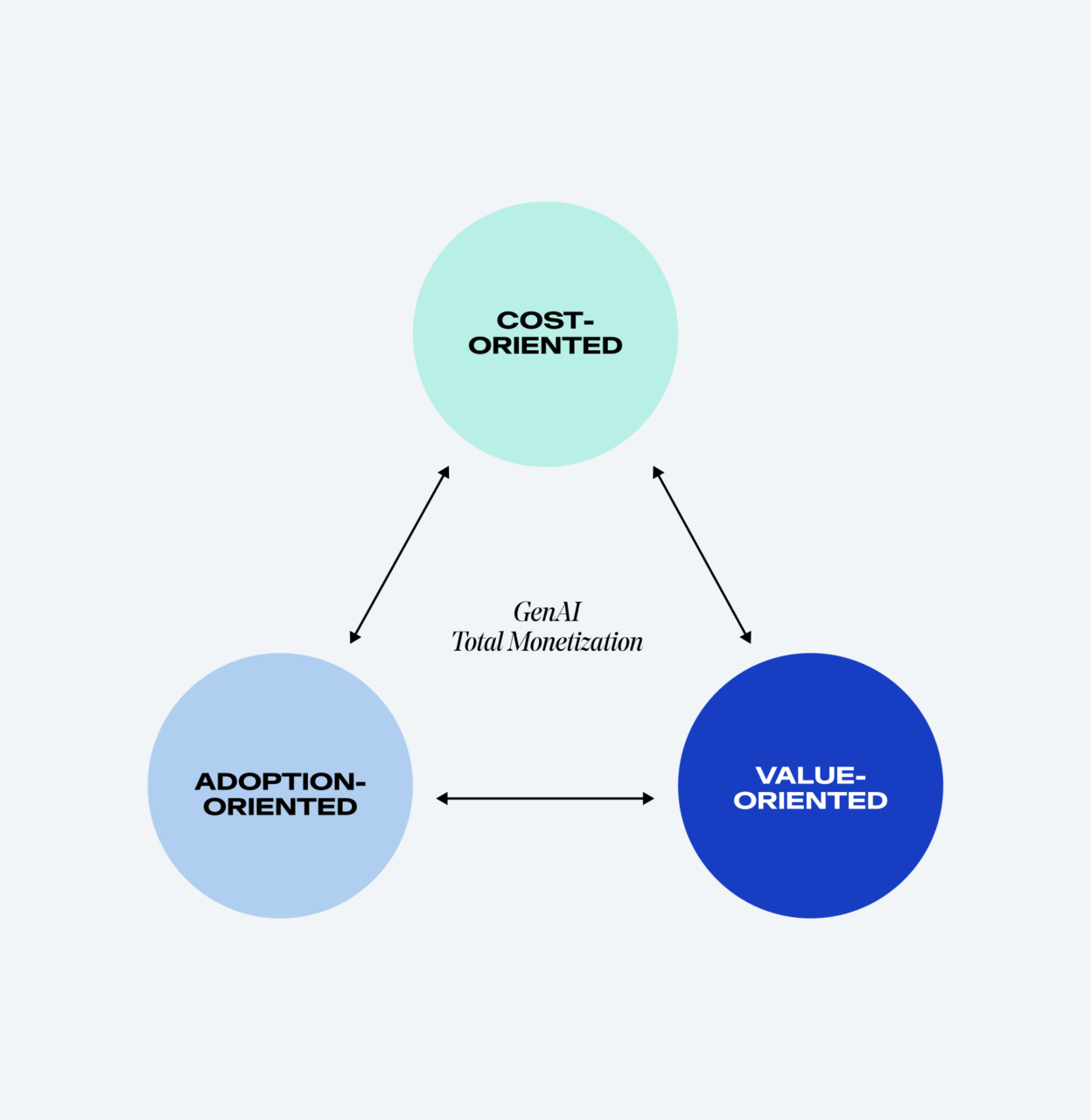
Image: Continuous iteration is required to balance cost, adoption, and value in order to achieve GenAI Total Monetization
While there is no “one way” to monetize for GenAI, understanding the interplay and challenges of these three key monetization focus areas can help provide strategy focus.
GenAI cost dynamics are dramatically different than traditional SaaS
In contrast to traditional SaaS, the very nature of GenAI solutions produces non-negligible costs for each individual user interaction or query, leading to much more dynamic marginal costs. Simply put, GenAI costs often scale with the number of user queries.
While Cost of Acquisition is one of the most important elements in SaaS economics, GenAI requires more scrutiny of the Cost of Good Sold. For example, OpenAI reportedly spends an estimated $700,000 daily to run ChatGPT. Even with the company surpassing $2 billion in annual revenue, operational costs are so massive that they still exceed revenue growth. And given massive demand for GenAI in the near future and increasing complexity in use cases, costs will likely not go down anytime soon.
Fueling these astronomical costs are, in part, the R&D required to create GenAI solutions: model (re)training, inference, and a limited number of AI experts, to name a few. Once operational, the recurring costs of hosting and running a solution, typically around 10% of revenues for a SaaS company, are significantly higher for GenAI (often by 5 to 15 percentage points).
Finally, each customer interaction with the underlying GenAI model, also known as inference, tends to have an associated non-negligible marginal cost, adding much higher cost variability into the unit economics mix. This differs from SaaS models, which benefit from stronger scaling effects.
As a direct consequence, more than a third of the 70 GenAI offers we assessed in this research are being monetized as a consumption (usage-based) model or as a hybrid model (combining elements of recurring and usage charges). By closely linking actual customer usage with pricing logic, such approaches help ensure a better alignment of revenue models with cost models. These offers are typically monetized based on activities or outputs such as tokens, CPU/GPU hours, images generated, etc.
This type of monetization approach is overrepresented for “underlying” platforms hosting GenAI models—such as OpenAI, Anthropic, Amazon, Cohere, Google Vertex, AI21, MistralAI, HuggingFace, and AWS Bedrock.
These usage-based and hybrid approaches are also now making their way into the higher-level “application” layer. For example, Microsoft charges for its Copilot for Security on a “per Security Computing Unit hour” basis. Adobe charges for its creative Firefly solution based on subscriptions that have a number of credits, while more credits can be bought.
Adoption is outpacing successful monetization
The rate of GenAI adoption and deployment has increased exponentially within enterprises over the last several months. GenAI players are betting that adoption will continue to increase. As employees and execs alike become more AI savvy, the use cases and related value potential could explode.
In fact, 91% of enterprise organizations expect productivity improvements from the use of GenAI.
In one-to-one conversations with executives and decision makers, especially from traditional SaaS players that have recently launched AI-infused plans, we’ve gleaned that adoption may be more restrained than many headlines suggest. However, it is still important for SaaS companies to get to market fast, despite the ballooning costs of GenAI mentioned previously.
In an effort to quickly launch their GenAI services, many companies are leaning on traditional SaaS approaches to monetization in order to capture revenue. These strategies aren’t likely to be sustainable in the long run.
What are some of the adoption-oriented trends we’re currently seeing?
A variety of SaaS-like trial options
More than 65% of companies studied currently offer free trials, limited either in number of days or number of interactions with the GenAI engine. This widespread mechanism in product-led SaaS sales allows potential customers to experience new technology firsthand, understand its value, and ultimately lower barriers to signup.
Over-reliance on traditional SaaS pricing models
In order to quickly launch and drive adoption, over 40% of offers that we analyzed rely on traditional SaaS models, such as per-user or per-seat pricing.This holds especially true for copilot-type offers, which are meant to augment existing users’ productivity. Examples include GitHub Copilot, Microsoft Office 365 Copilot, and Salesforce Einstein add-ons.
While generally acceptable, predictable, and easy to activate in the short term, per-user monetization models could become a thorn in one’s side in their journey towards a more sustainable business model. As a matter of fact, it drives an evident adverse effect: a highly active user (who should extract more value) is charged the same while being less profitable than one who uses the service more sporadically. In the case of Microsoft, they are reportedly losing 4x as much per active user in comparison to normal users.
To address potential drawbacks of this model and better balance costs with potential value generation, companies like Box are innovating by setting usage thresholds. For example, Box’s Enterprise Plus plan includes 20 GenAI queries per user/ per month, plus a company-wide pool of 2,000 additional queries, with options to purchase more as needed.
This capping strategy has been implemented by GenAI companies in terms of volume of interactions, as previously described, but also in terms of model speed, or model quality over a certain threshold. ChatGPT, Adobe Firefly, Google Gemini Business for Workspace or Regie Copilot also use such models.
Maximizing the potential of rapid adoption
All these points combined pose a unique challenge in aligning incentives between service cost and monetization. But how can companies progressively transition from risky adoption-focused strategies to more sustainable, value-focused models—especially as pressure is mounting?
The recent results from Adobe offer a perfect illustration. In a note to clients, Jefferies analyst Brent Thill wrote that AI will be “slower to monetize than investors want.” Adobe, Thill says, “continues to focus on driving AI adoption and usage.” This paves the way towards more innovative monetization models better aligning value creation and value capture.
Aligning pricing models to value
Fostering adoption using traditional SaaS user models does not seem to be a future-proof way for GenAI offers to cover the tech’s unique cost dynamics, let alone drive profitability. But a cost-oriented model might not enable buyers to properly predict or make sense of the price they’re being charged.
Instead of using purely cost-focused or adoption-focused strategies, companies should employ the same monetization best practices that drive recurring growth across industries: value-based monetization models.
A value-based approach focuses on setting prices primarily based on the perceived or estimated value of a product or service to the customer, rather than solely on the cost of production or market competition. As summarized in Prof. Oberholzer-Gee’s value stick, this helps align the price with the customer’s willingness to pay. This is why starting to monetize straightaway sends an important value signal.
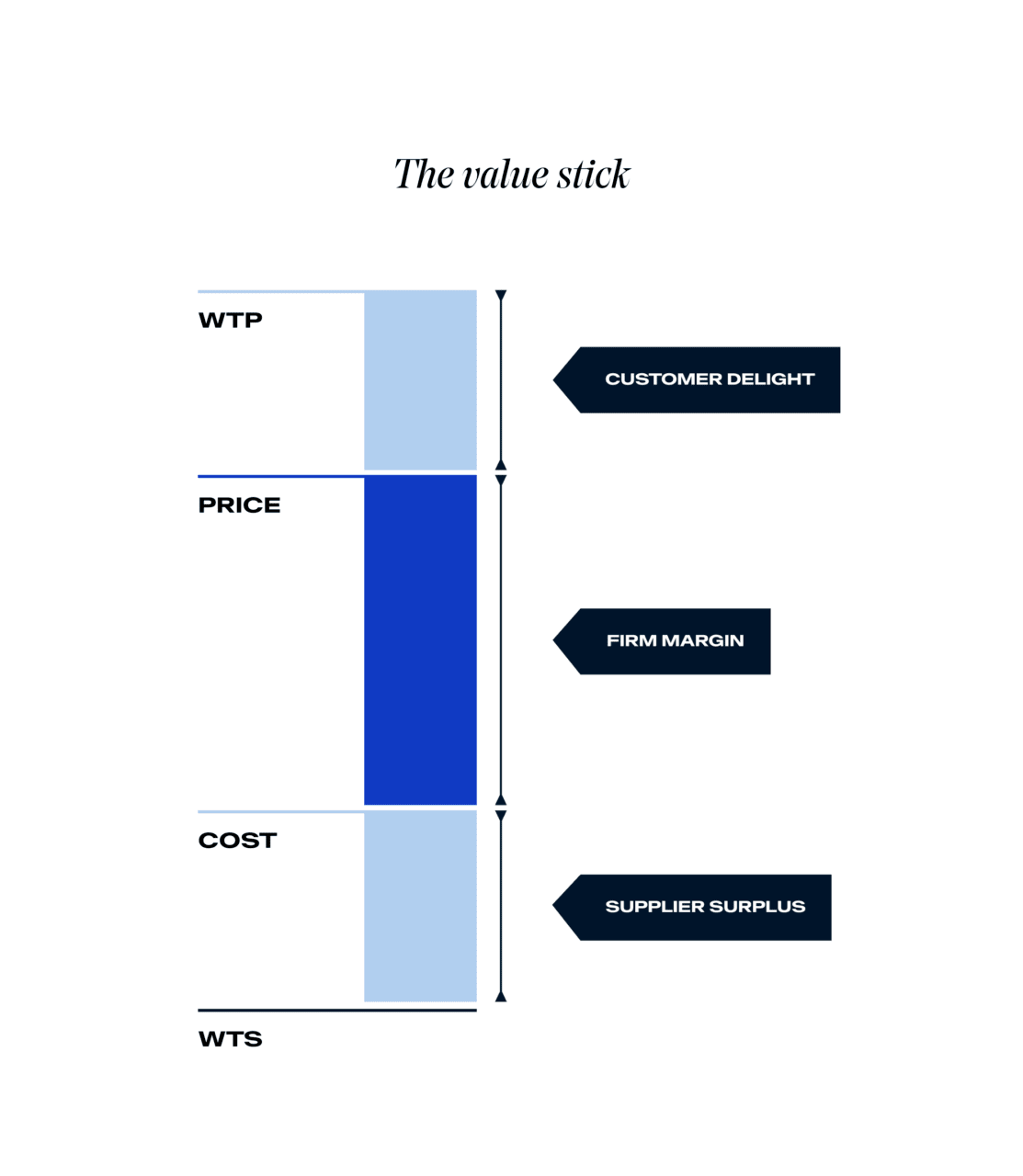
Source: Harvard Business School Online. WTP–willingness to pay; WTS—willingness to sell.
A value-based approach for GenAI
GenAI is still very new and radically different, so a value-based approach is easier said than done. Despite a clear understanding of the one-of-a-kind transformational nature of GenAI, many admit they are still clueless when it comes to properly monetizing their offer.
At the same time, customers are equally unsure when it comes to their willingness to pay for GenAI services. When asked what level of premium they’d be willing to pay for new or innovative GenAI features or capabilities, over 25% of execs responded that they simply “don’t know.”
Despite massive costs and risks, companies launching GenAI offers are forced into a real-time value exploration exercise. Now, success hinges on clearly understanding the value proposition of a GenAI offer to the customer and then quickly moving to align monetization with this. As a result, GenAI Mavericks have launched offers in beta stage to leverage the current hype, while hoping to drive enough adoption and data points to better explore optimal use cases, ideal customer profiles, and ultimately, measure value.
As an illustration, in August of 2023, it was projected that overwhelming operational costs would lead to bankruptcy for OpenAI by the end of 2024. However, the company moved quickly to scale monetization, leveraging probably one of the fastest ever run Price Sensitivity Meter Survey to help define their first price for ChatGPT.
Ultimately, just like other recurring services, GenAI can align on value and achieve profitability by learning from customer demands and the well established strategies of growth leaders.
Walking the value walk
With GenAI use cases evolving in real time, aligning on value can seem nearly impossible. Yet, many GenAI companies are already making strong value statements.
A GenAI offer, for example, may provide value by increasing employee efficiency and morale. GitHub has identified that its GenAI service Copilot helps developers code up to 55% faster, in addition to improving their overall job satisfaction. Intercom GenAI agent Fin resolves 30-50% of support questions instantly. Zoom Revenue Accelerator AI boosts business revenue and customers’ opinion of you by more than 20%. For small companies, content producing GenAI services like Canva or Jasper may help give them a marketing leg up that they could not have otherwise afforded.
This means that many GenAI offers are already “talking the value talk.” But in order to “walk the value walk,” they will need to capture and analyze data-driven customer insights in order to assess which value metric they are really impacting and how large that impact is.
Without substantiating those gains, it will be very difficult for an executive buyer to trust hundreds of GenAI vendors claiming significant efficiency or topline gains. Inundated with copilot offers promising 50% employee efficiency improvements, more than a few exec’s eyebrows are sure to start raising.
Consequently, proof of value can’t be limited to a simple theoretical exercise, but will require product managers and go-to-market teams to sit down with clients and listen, helping them progressively uncover value, assess it, and prove it. This proof-of-value, mutual discovery process benefits all parties involved. An example of this type of exercise can be seen in the paid pilots offered by Enterprise AI provider C3 AI.
Capturing the value premium
The following attributes can be predictors of the success of GenAI products in moving towards a more value or outcome-oriented model, thus capturing a higher value premium of the solution. In later articles in this series, we will take a closer look at the specific pricing metrics companies are using to achieve this.
Uniqueness of underlying data set | Exclusivity, depth and rarity of the data used to train the GenAI model, especially if the data set provides unique insights or competitive advantages. |
Specialization | Specialization in a specific domain or use case, addressing clear problems with high precision, making it more valuable to targeted customers. |
Attributability of value creation | Ability for the solution to be directly linked to quantifiable impact in terms of value creation and continuous return on investment (revenue growth, cost savings…). |
Time-to-value | Speed with which customers can deploy the solution and begin realizing tangible benefits, as quick wins support investment justification and prioritization. |
Scalability of solution | Ease with which the solution can be scaled elastically to accommodate growth in data, users, or complexity, affecting long-term value and adaptability. |
Regulatory and local compliance | Adherence to relevant regulations and data privacy measures, especially niche or local ones, minimizing legal risks for customers. |
Position in the GenAI value-chain | Criticality within the broader GenAI ecosystem. |
Offers in the top layers of the value chain, i.e. applications, are often in a more comfortable position to capture a value premium given their ability to leverage the aforementioned point. While many infrastructure and model providers will continue to most likely be facing a more commoditized and competitive situation—except for niche ones (e.g. hyperspecialization, ESG-friendly, etc.).
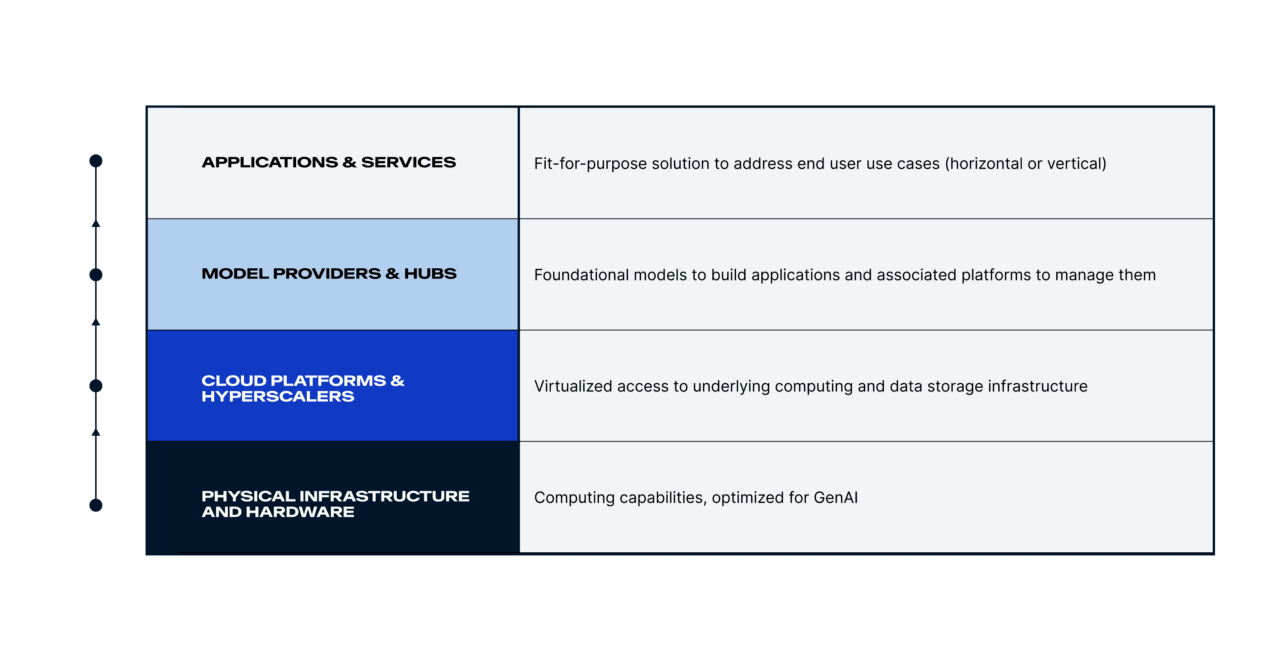
Image: 4-layer GenAI value chain. Some layers are merged for simplification.
In order to capture and share the value premium, companies should ideally employ a monetization metric that aligns with the value driver it optimizes. Or at a minimum, a metric that evolves in a similar manner. Additionally, use case and value exploration should examine the needs and usage of diverse personas.
The fact is, unsurprisingly, less than 10% of the 70 offers analyzed are actively doing this today in some shape or form (i.e. not “passing on” costs with a premium nor monetizing users). Intercom charges its AI chatbot Fin based on resolutions. At 11x, a company that provides go-to-market AI digital workers, 90% of customers are monetized via a “per task type” model, but the company is experimenting with outcome-based pricing, according to Kyle Poyar.
Ensuring that pricing metrics align with buyers’ value perceptions is also crucial. It fosters a sense of fairness and transparency. It demystifies the buying process by employing a customer-friendly metric, ensuring that their investment is justified by the value it delivers. And according to Subscribed Institute and Boston Consulting Group joint research customers considering GenAI solutions do indeed prefer usage/outcome-based pricing.
GenAI will be a long, continuous journey—not an overnight trip
Monetizing GenAI will be an evolving journey, demanding experimentation with pricing strategies and metrics. Currently, many companies start with simple metrics such as tokens or user counts, but over time, expect to see a shift toward more nuanced, outcome-based pricing models, closely tailored to specific use cases.
The evolution of these strategies will not be swift. Industry analyst Brent Thill predicts significant financial impacts from Adobe’s GenAI innovations won’t materialize until late 2024 to 2025, reflecting the slow, iterative nature of these adaptations.
Recent developments, such as GitLab’s introduction of its Duo Enterprise Tier and Microsoft’s launch of a global free trial for Copilot, illustrate the rapid changes and ongoing monetization experiments in the industry. Such dynamic adjustments highlight the importance of responsive pricing strategies that can quickly adapt to market demands and user feedback.
Ultimately, the aim of GenAI monetization is not merely competitive pricing but creating true value. Despite the pressures to cut costs and automate, the path forward is about aligning costs closely with value delivery. This approach will define the successful monetization of GenAI technologies, ensuring they are both sustainable and equitable.
Coming up next time
In our next installment, you’ll discover:
- A research-based analysis and examples of the 4 GenAI monetization avenues currently being used by leaders in the space
- The pros and cons of each avenue
- Which avenue nearly a third of GenAI companies are using and why
Turning GenAI innovation into revenue:
Learn the current top GenAI packaging models and assess how SaaS companies are using them today.
The 4 GenAI monetization avenues
Explore the leading positioning strategies of the pioneers in the GenAI monetization frontier—and the pros and cons of each.
Learn more about the author

EMEA Chair, the Subscribed Institute
Principal Director of Subscription Strategy, Zuora
The Subscribed Institute
