Propensity Modelling: How publishers ensure their subscription business keeps growing
What is a Propensity Model?
A propensity model is a specific type of statistical model used to predict the likelihood of a certain behavior or outcome based on historical data. In the context of digital publishing, a propensity model assigns a score to individual users, indicating their probability of engaging in specific actions, such as subscribing, renewing, or unsubscribing.
Key Components of a Propensity Model:
- Data Inputs: The model utilizes various data points, including demographic information, past behaviors (like article views and engagement levels), and interaction history (such as email clicks or responses). The quality and breadth of this data significantly influence the model’s accuracy.
- Algorithms: Propensity models can be built using different algorithms, ranging from logistic regression to machine learning techniques such as decision trees or neural networks. The choice of algorithm often depends on the complexity of the data and the specific outcomes being predicted.
- Score Assignment: After processing the input data, the model generates a propensity score for each user. This score quantifies the likelihood of the user engaging in a targeted behavior—higher scores indicate a greater likelihood of action.
What is propensity modelling?

How does it work?
Why is propensity modelling useful for digital publishers?
What types of propensity modelling can digital publishers take advantage of?
How does propensity modelling fit with segmentation?
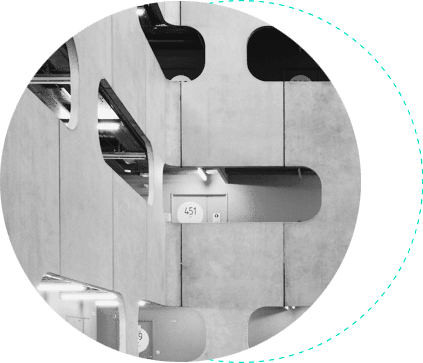
Challenges in implementing propensity models
While propensity modelling offers significant advantages for digital publishers, implementing it comes with its own set of challenges. Here are some common obstacles to consider:
- Data Quality and Availability: High-quality data is essential for effective propensity modelling. Publishers often struggle with incomplete, inconsistent, or outdated data, which can lead to inaccurate predictions. Ensuring that data is clean, comprehensive, and up-to-date is a crucial first step.
- Resource Constraints: Developing and maintaining propensity models requires dedicated resources, including skilled data analysts and robust technological infrastructure. Smaller publishers may find it challenging to allocate these resources effectively, potentially limiting their ability to leverage modelling.
- Complexity of Models: Propensity modelling can involve sophisticated algorithms and statistical methods. For teams without a strong data science background, the complexity can be overwhelming. This may lead to misinterpretations of the data or ineffective implementation of the models.
- Integration with Existing Systems: Successfully integrating propensity models into existing marketing and operational systems can be a logistical challenge. Publishers need to ensure that their CRM, marketing automation, and analytics tools can work seamlessly with the propensity models to realize their full potential.
- Privacy Concerns: With increasing regulations surrounding data privacy (e.g., GDPR, CCPA), publishers must navigate legal considerations when collecting and using customer data for propensity modelling. This requires a careful balance between personalization and compliance.
Best practices for successful propensity models
To maximize the effectiveness of propensity modelling, publishers should adopt the following best practices:
- Start with Clear Objectives: Define what you want to achieve with propensity modelling—whether it’s increasing conversions, reducing churn, or enhancing customer engagement. Clear goals will guide your model development and data collection efforts.
- Invest in Data Quality: Ensure your data is accurate, consistent, and relevant. Implement processes for regular data cleaning and validation, and consider investing in tools that enhance data quality.
- Segment Your Audience: Use propensity scores to segment your audience into meaningful groups. Tailor your marketing strategies based on these segments to increase relevancy and effectiveness.
- Continuously Monitor and Update Models: Propensity models should not be static. Regularly review and refine your models based on new data and changing user behaviors to maintain their accuracy and relevance.
- Leverage A/B Testing: Use A/B testing to validate your modelling assumptions and test different marketing strategies. This helps identify which approaches are most effective for different audience segments.
- Collaborate Across Teams: Foster collaboration between marketing, data analytics, and IT teams. A multidisciplinary approach can lead to more comprehensive insights and innovative strategies for using propensity modelling.
Key takeaways
FAQs About Propensity Models
Q: How do I start implementing propensity modelling?
A: Begin by defining your objectives, collecting high-quality data, and selecting the right tools or software. You may also consider collaborating with data analysts or data scientists for model development.
Q: What kind of data do I need for effective propensity modelling?
A: Key data types include demographic information, user behavior metrics (e.g., page views, time spent on site), and past purchase history. The more comprehensive your data, the more accurate your predictions will be.
Q: How often should I update my propensity models?
A: It’s recommended to review and update your models regularly—at least quarterly. This ensures they remain relevant as user behaviors and market conditions change.
Q: Can propensity modelling be used for more than just subscription services?
A: Yes, propensity modelling can be applied across various industries to predict customer behaviors, including e-commerce, retail, and service industries, enhancing marketing strategies and operational efficiencies.
Q: What are the potential ROI benefits of implementing propensity modelling?
A: By accurately predicting customer behaviors, publishers can enhance marketing effectiveness, reduce churn, improve customer retention, and ultimately drive revenue growth, leading to a higher return on investment.